Optimizing cancer therapy
Arka Roy focuses research on decision-making under uncertainty
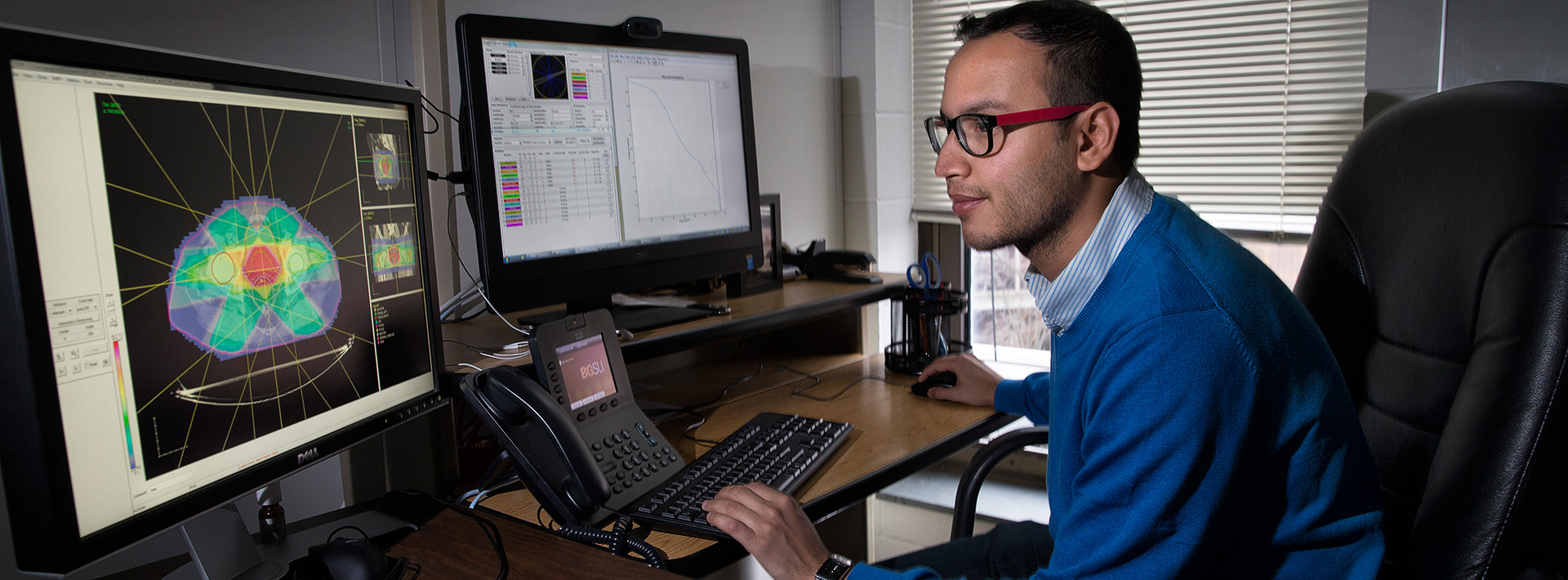
By Bob Cunningham
Arka Roy always wanted to be a medical doctor, but he was never too excited about medicine. Instead, he fell in love with mathematics.
It turns out, math proved to be the perfect tool for Roy to enlist in the fight against cancer. He joined Bowling Green State University as an assistant professor in the College of Business Administration in August after earning his Ph.D. at the School of Industrial Engineering at Purdue University. He focuses his research on optimal decision-making under uncertainty with applications in cancer radiation therapy.
“In basic terms, my search is not specifically for cancer research,” Roy said. “It deals with any kind of errors we have in our world, any kind of uncertainties we don’t know about. We come up with models that can encapsulate that uncertainty and include it in the models so that when everything under the assumptions of the model are met, you have optimal decisions. And when everything goes wrong and you have worst-case scenarios, you’re still within acceptable limits. So, we apply this whole concept into cancer therapy.”
Robust decision making, or robust optimization, is a large field beyond cancer therapy. It’s been around for some 50 years and it’s become prominent during the last 30 years.
“More and more people are using it in healthcare,” Roy said. “We see a very big prominent area in cancer therapy where it can really have a major impact. It’s a life-threatening disease and there are a lot of changes in patients’ bodies that are yet to be accounted for. There are a lot of uncertainties, so robust decision-making applies very well into that area.”
According to the American Cancer Society, there are expected to be 1.66 million new cases of cancer in 2016 and approximately 590,000 of those people will die from the disease.
The good news, Roy said, is that during the last 10 years the survival rate has increased 68 percent thanks, in part, to improvements in information flow and modern technology.
“These treatments can be categorized as surgery, chemotherapy and radiation therapy,” he said. “So, while these treatments have improved, there is potential to go further, much further. We come up with models to leap beyond such limitations.”
That means Roy is taking some of the guesswork out of cancer therapy. He looks at numerous changes that occur in a patient’s body, choosing one or two of them to try to tackle through the process of robust decision-making.
"About two-thirds of all people that get cancer get this radiation treatment type called intensity modulated radiation therapy, which is one of the more predominant types that we’ve used over the last four or five decades. During this time frame there are a lot of changes in the patient’s body. Tumors change in size and shape over time, so what we have come up with is a model that simulates the tumors’ change in size during that time-span.
“Tumors obviously shrink as a result of both radiation and chemotherapy and a variety of other factors, so we came up with a model that anticipates this change and plans out the radiation treatment over a five-to-seven week period. By anticipating this change, as well as multiple different scenarios, the radiation that’s delivered is still producing acceptable doses to the tumor and sparing healthy tissue and critical organs.”
Traditionally, Roy said, what happens is that these changes aren’t included in the ongoing therapy. After planning for the first treatment, the same pattern or treatment is repeated during a five-to-seven-week period.
Roy addresses radiation treatment in two ways.
“One is, we include tumor size changes, but then we show that just including it doesn’t solve the problem,” Roy said. “So, we take the guesswork out of it by anticipating multiple different scenarios or changes that can occur. Second, we attempt to come up with a treatment model that can incorporate all these different scenarios that may be realized.”
In this context, there are other sources of uncertainties.
“We looked at how tumors shrink over time and how we don’t know how it’s going to shrink exactly. I also looked at oxygen concentration in tumor cells. That actually plays a heavy impact toward the success of the treatment.”
Hypoxia, or low oxygen concentration in tissues, leads to a degrading treatment goal. The radiation doesn’t impact the tumor well enough if there is low oxygen concentration levels in the cells. The cells are monitored to help track any changes that may occur during therapy.
“If you take an image in the middle of treatment, how do we incorporate that?” Roy said. “How do we anticipate that image information right from the beginning of the treatment and how do we make it robust from the beginning?
"Mathematically, it really can be solved in numerous ways. One is anticipating a probability distribution over different scenarios and solving it over the different scenarios. Instead of looking at it as a probability distribution, look at it as an uncertainty set. That way, you always protect yourself against a very worst case. This is actually very effective if you’re looking at financial markets or healthcare—anywhere there is high risk and you want to protect yourself against the worst case. So we include these uncertainty sets into our optimization models.”
Updated: 12/02/2017 12:27AM